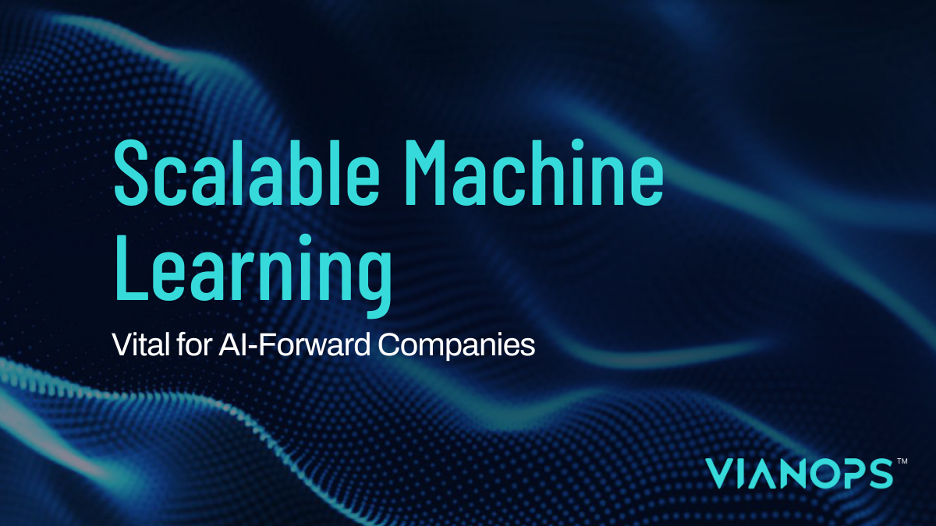
VIANOPS operationalizes reliability and performance of models at scale – without the high cost
By: Dr. Tao Liu, Vianai Systems
Machine learning algorithms built into models are commonly deployed in businesses to amplify output at a larger scale, automate processes, and make critical operational predictions.
With the advances in AI today, companies are adopting AI technology into R&D, product development, as well as business functions like sales and marketing, supply chain systems, HR tasks, and more, leading to millions or even billions of daily predictions by AI models across the company.
This is especially true for large, sophisticated, robust models running in financial institutions, payment processing companies, online marketplaces, CPG and retail companies, large manufacturing companies, and more. Progress with generative AI like ChatGPT is further accelerating AI adoption within enterprises.
While AI models are becoming larger and more complex with hundreds to thousands of features and millions to trillions of parameters, they still have many fundamental issues. Models are trained on specific datasets and when there are new or evolving, real-world scenarios, performance will begin to suffer, as well as the issues like bias & fairness, privacy, and security.
So how can AI-forward companies operate AI models continuously and ensure the health of the models in production?
The answer is scalability.
VIANOPS – High-Performance, Scalable AI Monitoring
VIANOPS is a purpose-built monitoring platform to enable high-performance, continuous operations. Scalability is the key design principle of VIANOPS across all the components from monitoring, root cause analysis, mitigation, to model validation and governance.
VIANOPS Scalability in Monitoring
-
- Monitoring a large number of models and setup monitoring for different complex models, which should be easy or fully automated.
- Monitoring large volume of inference data from thousands, millions to billions of inference data points at speed and low cost.
- Analysis and observation of data and model behavior from all different possible views, like different time windows and slices of data at different granularity.
VIANOPS measures changes in model input (feature data), output (predicted data), and ground truth data (actual) and determines where there are shifts in data. Users can set parameters for the percentage of variation that is acceptable. For example, if a model’s performance drops over 10%, an alert will be triggered and it will likely need to be retrained or replaced by a new model. When the retrained model or the new model performs better, the new model will replace the production model.
The evolution of ML monitoring has evolved from observing tabular data changes to emerging large language mode (LLM) monitoring. While LLMs are largely consumer-facing, more and more enterprises are harnessing the power of LMs for a variety of business applications like customer service chatbots, contract document understanding, and marketing content generation.
Every day, models receive streams of real-world data that may have new patterns that did not exist in the data with which they were trained. Nobody can guarantee that models will behave consistently when the world is changing so quickly, leading to a flood of new data points. Monitoring is critical to ensure models are healthy and trusted and behave as expected.
Monitoring LLMs is critical to detect and prevent issues in bias, and ethics, and requires scale to do so efficiently.
VIANOPS Scalability in Root Cause Analysis
The VIANOPS platform can slice huge amounts of data to surface the hotspots having statistically substantial shifts. It enables teams to identify variations in a way other models can’t as most significant changes can be buried and affect the ML’s output and predictions. We must preserve data to see how things have been changing in a macro way simultaneously with a micro view into a particular segment.
VIANOPS also enables users to observe the change of input and output over time in production with different time windows, like day to day, weekday to weekday, week to week, month to month, quarter to the same quarter last year, etc. This is essential to surface pattern shifts in short and long cycles.
VIANOPS looks at data from both a macro and micro lens to give users the best complete picture of how the model is running and what it’s producing. The platform monitors huge amounts of data points going beyond surface-level analysis and manages granular model monitoring.
VIANOPS Scalability in Validating and Governing Models
Validating and governing machine learning models at scale means the models are accurate, reliable, and follow ethical and regulatory standards. The data that goes into training ML models must be complete, correct, and consistent in order for the model to perform as it is intended.
As the model goes into production, it also must be validated. An MLOps platform should help data scientists and MLOps teams assess the model’s performance by evaluating the recent production data to ensure it meets the desired thresholds. Additionally, various risk metrics like data drift, data quality, bias & fairness, privacy, security and etc are to be analyzed to ensure risk compliance.
Automated tools like VIANOPS support manual reviews and human oversight from data scientists and MLOps teams to ensure successful validation governance at scale. The process is iterative, with continuous improvement based on feedback from real-world data and user input. By following robust validation and governance practices, organizations can build reliable and trustworthy machine-learning systems that provide value while minimizing risks.
The VIANOPS platform is unique in that it can run risk and performance analysis on a new model. By using real-time production data on the new model compared to the current model in production, VIANOPS can identify new risks and generate automatic reports with the right insights and recommendations to update and deploy the best-performing model.
VIANOPS Harnessing Scalable Machine Learning
VIANOPS is here for enterprises looking to scale machine learning operations affordably.
Traditional ML tools are not designed for the immense kind of scale we are dealing with in today’s machine learning models.
VIANOPS optimizes our tools to reduce the overall cost of our models with speeds 1,000 to 10,000 times faster than some popular, large-scale data processing tools at the same cost. This leads to more tangible business outcomes for those utilizing our tools and makes them more accessible.
Reach out for questions or if you would like to get in touch to learn more about how we can help your business’s ML operations or try VIANOPS for free, to experience scale first-hand.